Research
Introduction
Our eyes receive a constant stream of images from the visual world around us. The processing of this massive amount of information already starts in the retina, a neuronal tissue that lines the back of the eye and is part of the brain. The retina is not only a mere light sensor, it also performs a first analysis of the visual scene: It extracts and encodes potentially salient information to be forwarded to higher visual centers in the brain.
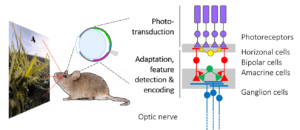
Our research aims at unravelling the sophisticated neural computations that take place in the retina. To this end, we present a diverse set of light stimuli to the explanted retina, while following the signals “trickling” through its synaptic networks using two-photon microscopy. From our work, we expect new insights into the function and organization of retinal circuits in the healthy and diseased retina – towards a better understanding of the underlying computational principles in the early visual system.
For some of the questions we are addressing, see videos (1, 2).
Research focus
All visual information from the retina to the brain travels along the optic nerve. However, due to its limited capacity, the optic nerve represents a major information bottleneck in the visual system. Therefore, before the transmission to the brain, behaviorally relevant features of the observed scene must be extracted and encoded. These include basic visual properties, such as brightness and color, but also more complex features like edges, object motion and direction.
What features are extracted is shaped – on the evolutionary scale – by the behavioral needs of an animal species (Baden, Euler, Berens 2019). For example, for a mouse, detecting a small dark moving object may provide potentially crucial information about food or danger depending on its position in the visual field: In the lower visual field, it may represent an insect on the ground and, hence, prey; in the upper visual field, it may indicate a predatory bird approaching in the sky. This simple example also illustrates that the context in which visual features appear, can be important.
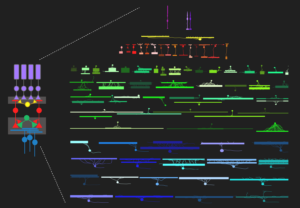
The retina’s computational abilities rely on likely more than 120 types of neurons organized in five distinct neuronal classes, which form complex circuits to allow the extraction of dozens of visual features (Baden, Schubert, Berens, Euler, 2018). Each feature is represented by a distinct type of ganglion cell. They are the retina’s output neurons; their axons form the optic nerve and send the visual feature representations in parallel to higher visual areas in the brain.
In our lab, we aim to elucidate the fundamental computational principles underlying early vision. Specifically, we investigate the functional organization of retinal circuits. We want to understand what visual information is encoded – in different parts of the visual field and especially in the context of the animal’s visual ecology (Qiu et al., 2022). In addition, we want to learn how diseases, such as progressive photoreceptor degeneration, impact the retina’s structure and function.
Methodological Approach
To address these questions, we established a comprehensive imaging approach for measuring neuronal activity driven by light stimuli along the retina’s entire vertical pathway using synthetic and genetically-encoded fluorescent activity sensors. Our key technique is two-photon microscopy, which enables us to excite fluorescent probes in the intact living retinal tissue using infrared laser light with minimal effects on the light-sensitive photoreceptor pigment (Zhao et al., 2020; Euler, Franke, Baden 2019; Euler et al., 2009). Therefore, we can simultaneously record activity in neurons at both population and subcellular levels, while presenting a range of light stimuli – from simple spots to natural color movies (Franke et al., 2019).
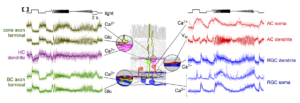
This approach is complemented by single-cell electrophysiology and immunocytochemistry. In parallel, we developed a comprehensive set of software tools, including stimulus presentation software and analysis pipelines for large-scale neural datasets. In addition, we started building retina models using deep learning (Höfling et al., 2022; Qiu et al., 2023; Korympidou, Strauss et al., 2024). These allow us to perform experiments in silico, such as efficiently exploring the vast visual stimulus space to derive qualitative predictions for targeted ex-vivo experiments.
In the future, we plan to build on our recent modeling efforts and “close the loop” by integrating online-analysis and model prediction in the biological experiments. Also, we will continue to tackle one of the big challenges of the retina: Understanding the computations provided by amacrine cells, with more than 60 types, the largest and most diverse class of retinal neurons.
Current Collaborations
Petri Ala-Laurila - University of Helsinki / Aalto University, Finland
Shahar Alon - Bar-Ilan University, Israel
Kevin Achberger, Stefan Liebau - University of Tübingen, Germany
Tom Baden - University of Sussex, Brighton, UK
Philipp Berens - Hertie AI, University of Tübingen, Germany
Matthias Bethge - AI Center, University of Tübingen, Germany
Kevin Briggman, Silke Haverkamp - Caesar, Bonn, Germany
Laura Busse - LMU Munich, Germany
Matthew Chalk - Institut de la Vision, Paris
Alex Ecker - University of Göttingen, Germany
Robert Feil - University of Tübingen
Katrin Franke - Standford University, USA
Olivier Marre - Institut de la Vision, Paris
Sebastian Seung - Princeton University, USA